WHITE PAPER
Improving Waterfowl Harvest Reporting: From HIP Surveys to Real-Time Solutions
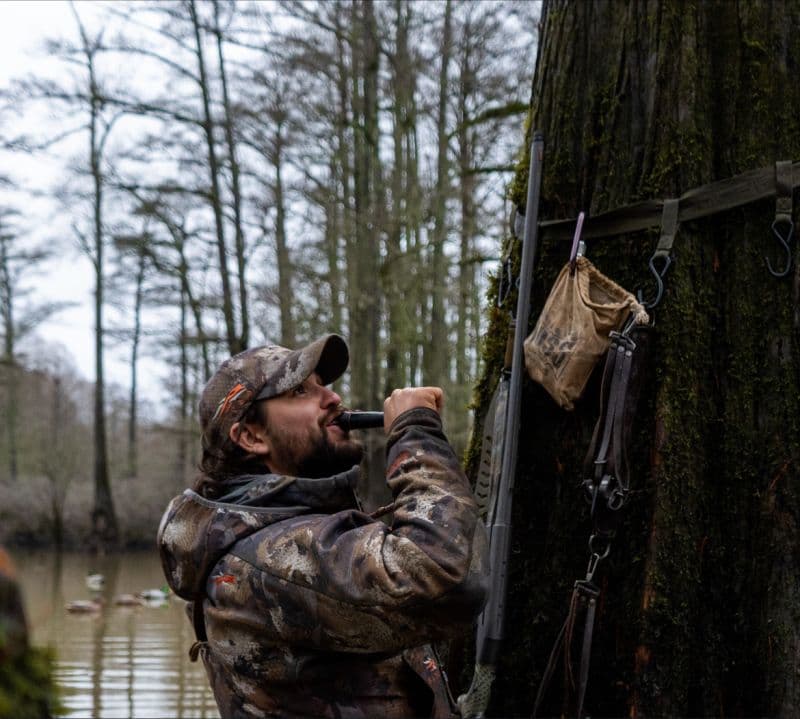
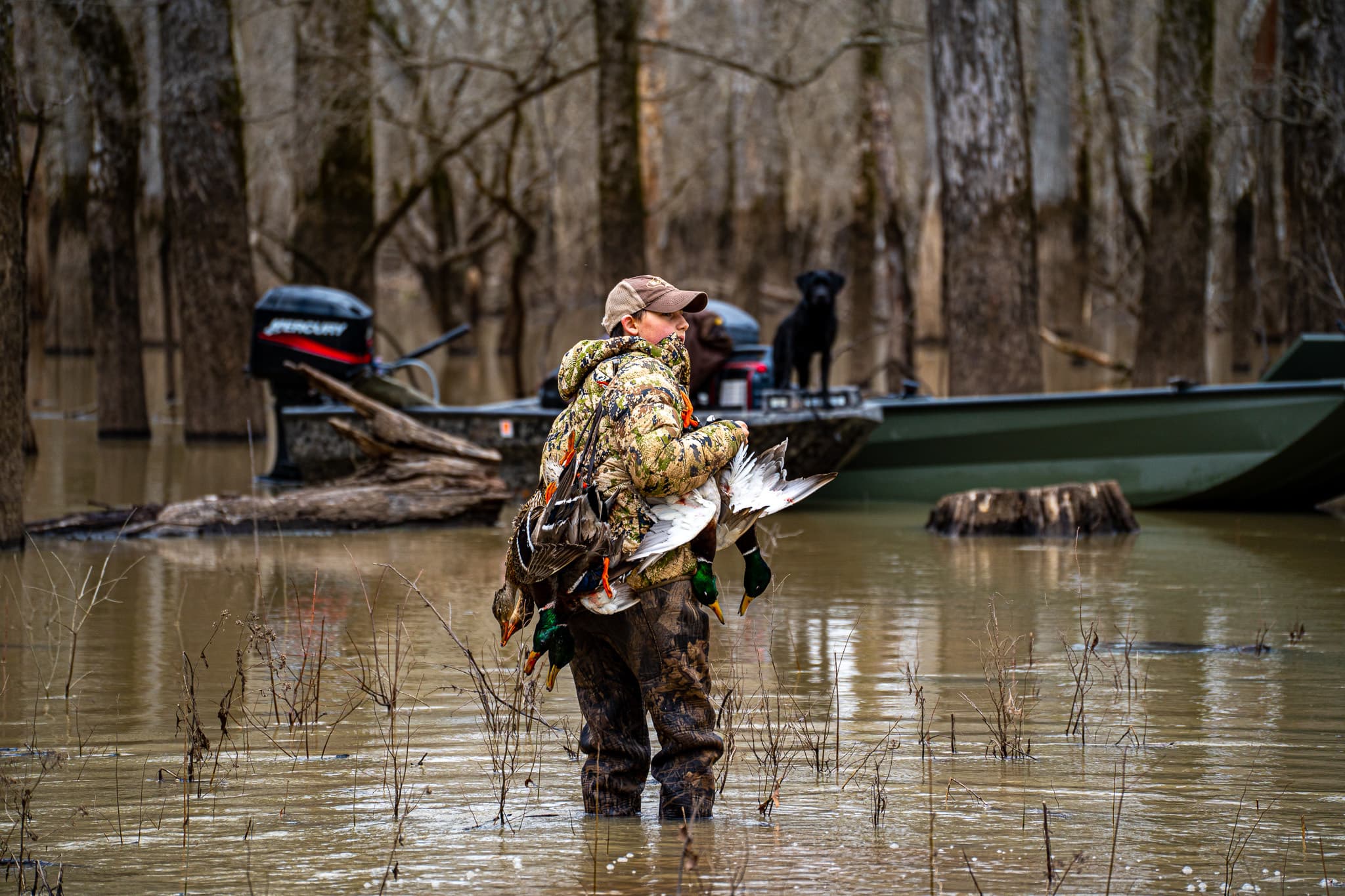
Introduction
Effective management of waterfowl populations relies on accurate data about hunter activity and harvest. In the United States, the primary mechanism for collecting waterfowl harvest information has been the federal Harvest Information Program (HIP), which surveys hunters after each season. Combined with the annual Breeding Population and Habitat Survey (BPOP) that tracks waterfowl populations each spring, these data inform hunting regulations and conservation efforts. However, current harvest reporting methods face significant limitations in timing and accuracy. This whitepaper examines the methods and issues of waterfowl harvest reporting via end-of-season HIP surveys – including recall bias, low response rates, and estimation uncertainties – and introduces GOVRAX: a geolocation-enabled mobile platform for real-time harvest tracking and wildlife reporting, draw system management, regulations querying, and a backend administrative portal to display all information. We explore how integrating GOVRAX into monitoring workflows can enhance data fidelity, reduce reliance on memory-based surveys, and better link harvest outcomes with population indices such as BPOP and nest counts.
Current Harvest Data Collection Methods (HIP Surveys)
Harvest Information Program (HIP): The HIP is a nationwide cooperative program between the U.S. Fish & Wildlife Service (USFWS) and state agencies, fully implemented by 1999 to improve migratory bird harvest estimates. All migratory bird hunters are required to register for HIP annually in each state they hunt, providing their name and contact information, and answering a series of screening questions about the previous year’s hunting success. These questions aim to identify what species groups the hunter pursued (duck, goose, dove, woodcock, etc.) and their success level, allowing USFWS to stratify hunters into sampling pools. For example, a hunter who reported >30 ducks last season would be categorized as “very active” for waterfowl, whereas a hunter who reported zero would be in a low-activity stratum.
Post-Season Surveys (Diary and Wing Surveys): HIP registration is just the first step in a three-part harvest survey process. After the hunting season, USFWS draws a stratified random sample of hunters from the HIP database to participate in detailed surveys. Selected hunters receive a Hunter Diary Survey (now often a web or mail survey) asking them to record the number of birds harvested and days hunted for the season. In addition, a subset may be asked to participate in the Parts Collection (Wing) Survey, sending in wings from harvested ducks or tail feathers from geese to help biologists determine species, age, and sex of the harvest. These two surveys – often referred to as the Migratory Bird Hunter/Harvest Survey (diary) and the Wing Survey – together provide the data to estimate total hunter activity and harvest by species across the country. It is important to note that the initial HIP screening questions themselves are not used to estimate harvest; they simply improve the efficiency and precision of subsequent surveys by ensuring representative sampling of active hunters.
Scale of Data Collection: Each year, tens of thousands of hunters are surveyed through this process. For example, recent federal information collection reports show roughly 67,500 hunters are contacted annually via HIP-based hunter surveys across all migratory bird categories. These surveys yield estimates of the total number of active hunters, total days hunted, and total harvest for each species group in each state and flyway. The results are published as annual Migratory Bird Hunting Activity and Harvest Reports by USFWS, which serve as the official record of waterfowl harvest for management purposes. While this system has been the backbone of harvest monitoring for decades, it is inherently retrospective – gathering data weeks or months after hunting activity – and depends on hunters’ willingness and ability to accurately recall and report their season.
Known Biases in HIP and Wing Surveys
Various forms of bias can distort the harvest estimates produced by HIP and the wing survey. Key known biases include:
Memory/Recall Bias: Hunters often misremember or forget their exact harvest when reporting after the season. This imperfect memory tends to cause undercounting of days when nothing was shot and over-reporting of birds harvested on successful outings. Early studies found that recall errors led to reported duck kills far exceeding actual counts (e.g., 56–168% above true harvest in some cases), making recall bias one of the largest error sources in hunter surveys.
Prestige/Exaggeration Bias: Some hunters over-report harvest intentionally, influenced by a desire to appear successful. This “bragging” effect (a form of response bias) can inflate the numbers reported. Together, memory lapses and prestige-motivated exaggeration are believed to systematically overestimate true harvest in self-reported surveys.
Nonresponse Bias: A significant portion of hunters do not respond to voluntary harvest surveys. If hunters who had poor success or did not hunt are less likely to respond (as studies suggest), the responders will be biased toward more active hunters. This leads to overestimation, since the sample is weighted toward higher harvests. For example, it’s thought that non-respondents tend to be less active/successful, so failing to account for them inflates the average kill per hunter. Recent HIP survey response rates are quite low (on the order of 18–28% in 2022–2023), raising concerns that nonresponse bias could be substantial today.
Coverage/Sampling Bias: The sampling frame for HIP must include all migratory bird hunters. If certain groups are missed or misclassified, harvest can be under- or overestimated. For instance, subsistence waterfowl hunters in Alaska (who don’t purchase standard licenses) historically were not covered by HIP, causing underestimation in those regions. Conversely, errors in how HIP registrations are collected have over-included some people: Many states found that license vendors issued HIP certifications even to hunters who never hunted migratory birds, inflating the roster of “hunters” used for surveys. One state (Arkansas) discovered that 65–70% of HIP registrants in recent years claimed to have taken zero migratory birds the previous year – an implausibly high fraction suggesting the frame included many inactive hunters. This kind of sampling frame error reduces the precision of estimates and can bias results if not corrected.
Sampling Error/Imprecision: Even if biases above are minimized, the HIP and wing surveys are based on samples and thus have sampling error. For groups with few hunters or low harvest, confidence intervals can be very wide. For example, in some states with very low goose harvest, the estimated take might be 2,900 geese ±120% (virtually +/- 3,500 geese!) due to small sample sizes. These large margins of error make it hard to detect true changes in harvest at finer scales. Overall, the precision of harvest estimates depends on getting enough representative responses, which is challenging when response rates are low and hunting activity is heterogeneous.
Empirical Evidence of Bias and Inaccuracy
Multiple studies have quantified the inaccuracies introduced by the biases above. Historically, controlled field studies were done to measure recall/response errors. For instance, researchers in the 1950s and 1970s compared hunters’ mail survey reports to actual bag checks in the field. They found large over-reporting biases: in one classic study, reported duck harvests were 53–168% higher than direct counts, whereas other errors (nonresponse + sampling) were much smaller (1.5–28%). The clear conclusion was that recall/response errors dominated the total survey error in those tests. In Canada, a similar experiment in 1968–70 showed that when including all hunters (even those who shot nothing), mail survey estimates overshot true harvest by about 60% on average; even when looking only at successful hunters, reports were ~16% high. These early empirical studies cemented the notion that voluntary hunter reports tend to overestimate actual kill, mainly due to memory and nonresponse factors.
More recently, modern analytical studies have reinforced these findings. A landmark analysis by Padding & Royle (2012) compared the U.S. Fish & Wildlife Service (USFWS) harvest survey estimates to an independent benchmark: band recovery data. They examined banded mallards and Canada geese – for which the true harvest can be estimated from the number of banded birds shot (adjusting for reporting rates). The results showed a striking overestimation by the surveys: the survey-based duck harvests were on average 1.37× higher than the band-based estimates, and goose harvests were 1.50× to 1.63× higher (depending on time period/method). In other words, the traditional HIP/mail surveys appeared to overshoot actual duck kill by ~37% and goose kill by 50–63%. The authors derived correction factors: They suggest multiplying published duck harvests by 0.73 and goose harvests by 0.61–0.67 to get closer to reality. This is a dramatic validation that systematic overestimation exists in the national harvest statistics. Notably, the bias for geese was even greater in the HIP era (post-1999) than under the old pre-HIP survey, implying changes in survey methodology or hunter behavior might have worsened the over-count for geese.
Field experience from wildlife agencies supports these conclusions. State biologists have long suspected that some HIP data are unreliable because of hunter response issues and license vendor practices. For example, if a license agent hands every buyer a HIP number without ensuring they answer the screening questions, the sample frame gets cluttered with people who didn’t actually hunt migratory birds. This reduces the efficiency and accuracy of subsequent surveys. The Wildlife Management Institute reported in 2021 that in states like Louisiana and Arkansas, many HIP registrants “had not hunted migratory birds in the previous year,” indicating HIP registration totals were inflated with inactive hunters. After those states reformed their process (requiring online or in-person HIP signup where the hunter must actively answer the questions), the number of HIP registrations dropped to “in line with expected numbers,” and data quality improved. In short, cleaning up the sample frame by removing non-hunters is expected to reduce overestimation bias (since previously many “zero” hunters likely never responded, skewing the sample toward active hunters).
Another area of empirical evaluation is response rate and nonresponse bias in recent surveys. The USFWS has been transitioning its Migratory Bird Hunter Survey to new online systems, and reports publish the response rates. For the 2022–23 season, only about 18% of sampled hunters responded; for 2023–24, about 21% responded. This means nearly 4 in 5 hunters did not send back any harvest report. If indeed many of those nonrespondents harvested little or nothing (a reasonable assumption), the estimates based on the 20% who did respond will overestimate average kill per hunter. The FWS explicitly acknowledges this potential “differential response” issue – they note that changes in the survey (described below) might be “affecting response rates and non-response bias (differential response rates of hunters who hunted and did not hunt)”, and they plan additional studies to evaluate it. A 2019 study from France underscores how critical this is: it argued that the main cause of overestimation in bag surveys is nonrespondents with zero harvest; through simulation, the authors showed that if you can push final response close to 100% (by repeated follow-ups), you largely eliminate this bias. In fact, when they forced a near-100% response in their model (by a last-phase phone interview of all remaining nonrespondents), the overestimation disappeared and the estimates became unbiased. This highlights that improving response rates is key to accuracy – a point U.S. managers are well aware of.
Regarding the wing (Parts Collection) survey, empirical tests have mostly focused on identification accuracy. As mentioned, a controlled experiment in 2002–2004 sent known-age duck wings to the flyway “wingbee” panels and then checked their determinations. The good news is species ID and sex were almost perfectly accurate (>99% correct), so the survey gives a reliable species breakdown of the harvest. Age classification was more variable by species: for mallards, ~95–96% of wings were aged correctly (adult vs juvenile), but for a small duck like blue-winged teal, accuracy was only ~83–84%. The analysts found that for blue-winged teal, this aging error rate would cause unacceptably biased age ratios in the majority of wing surveys (~69% of years). In practical terms, that means the juvenile-to-adult ratio reported for teal harvest might be off in many years, which could mislead assessments of annual production. Mallards and wood ducks, on the other hand, had enough accuracy that the age ratios from the wing survey were deemed “insignificantly biased”. Thus, the wing survey is quite solid for species composition (critical for setting species-specific bag limits) but has some limitations in age structure data for certain species. Participation bias in the wing survey (who sends in wings) is harder to measure; however, since wing survey participants are drawn from those who responded to the diary survey, it likely overrepresents more dedicated hunters. The FWS mitigates this by weighting the wing data according to each state’s harvest, but if some hunter types (e.g. casual 1-2 day hunters) rarely send wings, their harvest might be under-sampled in species composition. No recent publications directly quantify this, but it’s a recognized consideration in survey design.
In summary, a wealth of evidence shows that the HIP/diary survey tends to overestimate total harvest (on the order of ~30–60% too high) due to recall and nonresponse biases. The Parts Collection (wing) survey improves species data quality but is not immune to error, especially for certain species’ age data. These biases are not just theoretical – they have been measured in the field and via analytical comparisons. This has prompted exploration of improved methods, both within the U.S. and internationally, to obtain more accurate and timely harvest data.
Figure 1 below illustrates bias: the orange bars (based on band recovery “true” harvest) are considerably lower than the survey-reported harvest (yellow bars) for both ducks and geese. Such biases, if uncorrected, can mislead management analyses (for example, overestimating harvest rate or inferring unrealistically high population removal by hunters).7
Figure 1: Apparent overestimation of waterfowl harvest in HIP surveys, as revealed by a comparison with band recovery data. Surveys reported ~37% more mallard ducks and ~63% more Canada geese harvested than were indicated by independent band-based estimates. This suggests substantial bias in recall-based, voluntary harvest reporting.
Linking Harvest Data with Population Surveys (BPOP and Beyond)
Accurate harvest reporting is not only important on its own, but also in conjunction with population monitoring programs. The Waterfowl Breeding Population and Habitat Survey (WBPHS), commonly known as BPOP or the May Survey, is the largest wildlife survey in the world, covering over 2 million square miles of prime breeding habitat across the U.S. and Canada. Since 1955, BPOP has provided annual estimates of duck populations and breeding conditions, and it has guided waterfowl harvest and habitat management decisions. Harvest data from the previous fall, together with BPOP spring counts, are key inputs to Adaptive Harvest Management (AHM) models and other frameworks that set hunting regulations. In AHM, for example, managers use population size estimates (from BPOP) and harvest estimates (from HIP) to adjust season lengths and bag limits in a way that optimizes long-term sustainability. Reliable harvest figures are needed to calculate harvest rates, assess the impact of hunting on populations, and calibrate population models (such as the Lincoln estimator, which uses band recovery and harvest data to infer total population size).
When harvest estimates are biased or imprecise, they can cloud our understanding of population dynamics. The Padding & Royle study noted that while the historical overestimation in harvest surveys likely did not cause mismanagement (since harvest regulations tend to be conservative and informed by multi-year trends), it “has negative impacts on some applications of harvest estimates, such as indirect estimation of population size.” In other words, if we think more birds are being shot than genuinely are, we might underestimate actual population size or survival rates when using those data in models. Conversely, any under-reporting of harvest could mask potential overharvest issues. Thus, fidelity in harvest data is critical for sound science.
Furthermore, emerging issues in waterfowl management, such as monitoring the effects of climate change on migration timing or detecting regional declines in certain species, require integrating data from multiple sources. Harvest trends, hunter effort distribution, and habitat conditions must be seen together. If harvest reporting could be more real-time or fine-grained, managers could, for instance, detect a mid-season drop in harvest in a particular region and investigate if it correlates with a sudden freeze or habitat loss. They could also better correlate nesting success and brood observations with the subsequent fall’s harvest. Currently, agencies conduct separate nest counts and brood surveys on summer breeding grounds to gauge reproductive success. However, these are often limited in scope. Additional crowd-sourced data on waterfowl sightings (pairs, broods, migrants) from observers on the ground could greatly enrich the picture. Ideally, harvest data and wildlife observations collected in the field by hunters should complement the formal surveys. This synergy can improve our understanding of how harvest pressure and population status interact. For example, if an area shows very low harvest and many hunter reports of “no birds seen,” and then BPOP finds a population dip, those data together strengthen evidence of a true decline. Conversely, real-time field reports of abundant juveniles in the fall (indicating a good production year) could help validate BPOP’s spring prediction of a bumper crop of ducks.
In summary, the current paradigm gives us a broad but delayed view: we get annual population indices (BPOP) and annual harvest estimates, and we look retroactively at how they align. To advance waterfowl conservation, we should strive for more timely, continuous monitoring where harvest data collection keeps pace with ecological changes. This is where technological innovation like the GOVRAX platform can play a transformative role by modernizing harvest reporting and integrating it with real-time field observations.
Impacts of Survey Flaws on Management Decisions
The ultimate concern is how these reporting inaccuracies affect waterfowl management. Harvest data are used for multiple management purposes: setting annual hunting regulations (season lengths and bag limits), assessing harvest pressure on populations, modeling survival rates and population size, and understanding hunter participation trends. Biased or imprecise data can lead to suboptimal decisions, but managers also account for uncertainty in various ways. Here are some key insights on the management implications:
Setting Bag Limits and Season Frameworks: For most North American duck species, hunting regulations are determined through Adaptive Harvest Management (AHM), which relies on population surveys (breeding counts) and harvest rate estimates (from banding studies) more than raw harvest totals. This was intentional – AHM was developed in the mid-1990s when it was acknowledged that harvest survey estimates have noise and bias. Thus, waterfowl managers have historically been cautious about using the absolute HIP harvest numbers to make year-to-year regulatory changes. The 2012 banding comparison study noted that the longstanding overestimation in harvest surveys “likely has not influenced waterfowl harvest management policy in the USA”, precisely because harvest strategies were built to be robust to such errors (e.g., they focus on trends and rates rather than point estimates). In practical terms, this means that even if, say, the HIP survey said 15 million ducks were shot one year but the true number was 11 million, the regulatory prescriptions (liberal/moderate/conservative package) didn’t change, since those are driven by breeding population size and allowable harvest rates, not the survey’s harvest total. However, for specific species harvest strategies, biases can matter. For example, American black ducks and Atlantic population Canada geese have quota-based or target harvest frameworks that do incorporate harvest estimates. If those estimates are inflated, managers might set unnecessarily restrictive limits. Recognizing this, agencies have sometimes applied ad-hoc “adjustments.” In the case of black ducks, for years the harvest was thought to be higher than it actually was (later analysis showed reporting rates and survey bias meant true kill was lower); managers cautiously kept bag limits low, which in hindsight was extra-conservative but not harmful. If the bias had been in the opposite direction (underestimating harvest), we could have seen over-harvest without realizing it – a more dangerous scenario for the population.
Estimating Population Size (Lincoln estimator): One important use of harvest data is the Lincoln estimator, which provides a cross-check on waterfowl population size by using band recovery data and total harvest. Essentially, Population = (Total Harvest) / (Harvest Rate). Agencies often estimate harvest rate from banding (e.g. % of the fall population that is harvested, based on band returns) and plug in the survey’s total harvest to estimate population size. If total harvest is overestimated by 30–40%, then the calculated population would also be overestimated by 30–40%. This could lead to false confidence about the size of the waterfowl population. Padding & Royle (2012) explicitly warned of this: the apparent bias in harvest surveys can “have negative impacts on applications such as indirect estimation of population size,” and they recommended applying the correction factors (0.73, 0.61, etc.) to harvest estimates before using them in such analyses. For example, using uncorrected harvest data in a Lincoln estimator might suggest a mallard breeding population a few million higher than reality – potentially skewing management planning (like habitat or harvest goals). By adjusting harvest down to account for bias, managers get a more accurate read on population status.
Harvest Rates and Survival Analyses: Waterfowl survival and harvest rates are typically estimated from banding programs, which are thankfully not dependent on the HIP harvest survey. Banding analysis uses the fraction of bands shot (and reported) to infer harvest rate directly. Thus, survival modeling has been insulated from HIP bias to a large extent. However, in the absence of adequate banding, one might try to use harvest survey data to approximate harvest pressure on a species. For less-common species or those not heavily banded, survey overestimation could mislead managers about how big a toll hunting is taking. For instance, if HIP says 50,000 wood ducks are harvested in a state, but true number is 35,000, managers might think harvest rate is higher relative to the population than it actually is, possibly prompting unwarranted regulation changes. On the flip side, if bias were ever to swing (say a new reporting method suddenly cuts over-reporting), the apparent drop in harvest could be misinterpreted as a decline in hunters or birds when it’s really just more accurate data. That’s why consistency in methodology has been important for detecting trends.
Trend Detection and Management Response: One fortunate aspect is that many biases (memory, prestige, even some nonresponse) are somewhat systematic – meaning they likely occur each year at a similar magnitude. While they inflate the absolute numbers, they might not completely obscure trends (year-to-year changes) as long as the biases don’t worsen or improve over time. Managers primarily look at trends in harvest (increases or decreases) as one indicator of how regulations and populations are interacting. If the overestimation bias is relatively constant, the trend (up or down) in the raw numbers is still meaningful. However, if something causes the bias to change (e.g., a big drop in response rate over the years, or a shift to online reporting that cuts down exaggeration), then an observed trend might be partly an artifact. For example, HIP harvest estimates for geese jumped in the late 1990s compared to the prior survey method – this was largely a methodological change, not an actual huge jump in goose kill. Managers had to recognize that and not overreact to the raw numbers. Similarly, the USFWS in 2023 is being cautious interpreting harvest trends until they evaluate how the new survey methods affect bias. Once they quantify that, they can adjust and continue comparing to previous years appropriately. In general, harvest data is one piece of the puzzle in setting regulations; population surveys (like the May Breeding Waterfowl Survey) carry more weight for ducks. Thus, the biases in harvest reporting, while real, have not drastically derailed harvest management to date. Managers have treated the HIP estimates as estimates (with error bars and potential bias) rather than gospel.
Game species-specific decisions: For some migratory game birds (doves, woodcock, etc., which are also surveyed via HIP), the harvest estimates are a primary management metric since those species may not have extensive banding or independent population surveys. In such cases, biases could be more directly influential. For example, if the HIP survey is overestimating mourning dove harvest by 30%, managers might believe hunter success is high and populations can sustain current pressure, when in reality harvest might be lower (which is actually safer for the population, though it could also mask a decline in hunter activity). On the flip side, if response rates declined and led to under-representation of high-volume hunters, the survey could underestimate harvest, potentially leading managers to increase opportunity thinking harvest is low. These scenarios underscore why ongoing evaluation of the survey’s accuracy is critical – agencies periodically validate the estimates (through banding or special studies) to ensure they’re not drifting into unreliable territory.
Adaptive management and fine-tuning: Flaws in data ultimately reduce the precision of management. If estimates are noisy or biased, it’s harder to detect real changes in waterfowl abundance or the effects of regulation changes on harvest. This can slow the adaptive management feedback loop. For example, if a liberalized bag limit only modestly increases actual harvest, but the survey exaggerates that increase, managers might incorrectly conclude the regulation had too large an impact and scale it back. Conversely, if the survey failed to show an increase when one occurred (due to sampling error), managers might miss an opportunity to adjust. Better data = more confident decision-making. This is why the USFWS and states are investing in improving HIP data quality – “better quality data will help improve harvest management”, as one FWS official noted, and will also assist in evaluating hunter recruitment and effort initiatives. In management meetings, biologists have explicitly discussed the need to address recall bias, nonresponse, and timeliness so that harvest estimates can be used more directly in decision frameworks (for instance, in refining harvest strategy models or setting more responsive quotas).
Alternative and International Reporting Systems
Recognizing the limitations of traditional hunter surveys, agencies have looked at alternative approaches to harvest reporting. Around the world, there is a spectrum of methods used for migratory game birds, ranging from enhanced surveys to mandatory reporting systems:
Canada’s National Harvest Survey: Canada runs a system analogous to HIP. All migratory bird hunters must purchase a Canadian Migratory Game Bird Hunting Permit (which acts as a federal duck stamp), and a portion of them are mailed a survey about their hunting activity. This provides a known sampling frame (all permit holders), avoiding major coverage gaps. However, the Canadian mail survey historically suffered the same recall and nonresponse biases as the U.S. surveys. The Canadian Wildlife Service in the late 1960s–70s also found over-reporting of harvest on mail questionnaires, as discussed earlier (up to ~60% over true kill when including all hunters). In recent years, Canada has moved to online reporting options and sends reminders to improve response, similar to U.S. efforts. The advantage Canada has is a true “mandatory” permit: they know exactly how many migratory bird hunters there are (because you cannot legally hunt waterfowl without buying the federal permit). This means no underestimation from unlicensed hunters (whereas in the U.S., a small risk exists if someone hunts without HIP). But it does not automatically solve response or recall bias. Essentially, Canada and the U.S. have been learning in parallel how to refine survey-based estimates – for example, both countries hold annual “wing bees” to identify harvested birds’ species, and both face declining response rates in the internet age, requiring new strategies.
Mandatory Reporting Systems (International): Some countries have implemented compulsory harvest reporting for hunters, which can greatly reduce nonresponse issues. A notable example is Denmark, where all licensed hunters are required by law to report their annual game harvest (including waterfowl). Denmark’s system is considered “well-functioning,” achieving a 96% reporting rate each year. Hunters log their kills (originally via mail, now increasingly online), and any anomalous data is immediately checked by authorities by following up with the hunter. Denmark is even launching a mobile app to make harvest reporting more convenient, further modernizing the process. The result is a near-census of harvested birds in Denmark, available to managers within a couple of months after season close. Other European countries have mixtures of voluntary and mandatory systems. For example, Finland requires reporting for certain species or areas but not others, and has noted issues with hunters sometimes mis-recording migratory birds as local species. France and some others historically relied on voluntary surveys, but there is growing interest in more rigorous reporting to enable flyway-level management of shared migratory species. In the UK, harvest reporting for waterfowl is largely voluntary (through hunter diaries or membership organizations), which likely undercounts total take. The European Union’s Birds Directive now asks member countries to report national harvest numbers for each species, which has put pressure on countries to improve data collection (many are turning to hunter portals or apps to gather these stats). Overall, the trend internationally – especially for migratory waterfowl that cross borders – is toward more standardized and comprehensive reporting, often leveraging technology.
GOVRAX: A Real-Time, Geolocation-Enabled Reporting Platform
GOVRAX – short for “Government Recreation Access” – is a new modular software system designed to bring modern technology to wildlife harvest reporting and public land access management. Founded by passionate outdoorsmen who are also U.S. Special Operations veterans, GOVRAX embodies a mission-oriented approach to conservation challenges. The platform provides a one-stop mobile solution to manage hunting access, collect real-time harvest data, and visualize historic user location information to understand high-pressure areas. In essence, GOVRAX turns the traditional paper-based check-in and harvest survey process into a seamless digital experience: hunters use the app or an NFC-enabled hard card to check in and out of hunting areas, log their harvests on the spot, and even report wildlife sightings, all with geotagging and timestamping. Key features include:
Real-Time Check-In/Check-Out: Hunters can use GOVRAX to check in when they arrive at a public hunting area or leased property and check out when they leave. This creates an automatic log of hunting effort – who is hunting where, when, and for how long – information that is valuable for managing hunter distribution and measuring effort. Real-time check-in also allows for capacity management on popular public lands (preventing overcrowding by showing how many users are already in the field) and enhances safety (managers know if someone hasn’t checked out by the expected time). The data the check-in/out process yields also contributes to understanding nonresident vs. resident hunter density on public access areas. Because the app leverages GPS, it works even in remote areas with poor cell coverage; data is cached and uploaded once connectivity is available. This offline capability is crucial for backcountry use.
Location-Based Harvest Reporting: While in the field (during the hunt or immediately after), a hunter can “Mark Harvest” in the app, logging each animal taken. The app interface guides the user to input details such as species, sex, and other relevant attributes (for example, for a duck harvest, they might log “Mallard – Drake (4), Green-Wing Teal - Drake (2)” or for a deer, “White-Tailed Deer – Buck – Rifle – 8 Points”). The harvest entry is geo-tagged to the hunter’s location and timestamped. This approach virtually eliminates recall bias – the data is recorded at the moment of harvest, not hours or months later. It also ensures high-resolution data: instead of just end-of-season totals, managers can see each individual harvest event with time and place. This is akin to every hunter keeping a daily diary automatically. Over the season, the app builds the hunter’s personal harvest log (and these can be aggregated for population-level analysis).
Wildlife Sightings and Observations: GOVRAX isn’t limited to reporting harvested game. The platform encourages users to log wildlife sightings as well. For instance, if a hunter in a duck blind observes dozens of pintails landing in the marsh or notes seeing a rare species, they can record that information. These sightings (with location and date) create a rich dataset of wildlife presence and distribution, far beyond what harvest numbers alone show. Over time, a network of hunters reporting observations could function similarly to citizen-science birding databases, but integrated with hunting activity. Such data could be used to track migration progress (e.g., users reporting the first snow geese arriving in their area), habitat use, or even nesting activity (turkey hunters in spring could log hens and poults seen, waterfowl hunters could report banded birds sighted, etc.). This contributes to a broader picture of ecosystem health that agencies can utilize.
Integrated Access Management: True to its name, GOVRAX also streamlines access to public and private lands open for hunting. Users can discover available hunting areas (including Walk-In or Block Management lands, which often require signing in), obtain permits, and navigate property boundaries via in-app maps. From an agency perspective, this means harvest reporting is directly tied to land management. For example, a state wildlife agency could require hunters to check in via GOVRAX for certain wildlife management areas – once checked in, the app can automatically prompt harvest reporting at checkout. This tight integration could yield near-complete reporting compliance (since the act of checking out forces a yes/no declaration of any harvest). It effectively makes reporting a built-in step of the hunting outing, rather than a separate voluntary survey weeks later. Additionally, the geolocation aspect prevents the common problem of ambiguous data – the system knows exactly which unit or county the harvest came from without relying on the hunter’s memory or knowledge of zone boundaries.
Veteran and Outdoorsman Ethos: The developers of GOVRAX bring a user-centric and mission-driven ethos. As a service-disabled veteran-owned small business, the company’s leadership (former Army Ranger Justin Neal and former Navy intelligence analyst Taylor Cassat) is comprised of avid hunters who understand the hunting community's and conservation officials' needs. This has shaped the app to be user-friendly in the field (even for those not tech-savvy) while also meeting the data requirements of wildlife agencies. The platform also emphasizes privacy and security so that hunters can trust that location data is handled responsibly and used for conservation purposes, much as they have trusted duck band returns and surveys in the past. By coupling a community-minded approach (connecting like-minded outdoor enthusiasts) with high-tech data collection, GOVRAX aims to increase hunter engagement in conservation science.
Real-Time Data Dashboard: On the backend, GOVRAX offers agencies a live dashboard of harvest and effort. Instead of waiting until next year’s report, a waterfowl program coordinator could see daily updates of how many ducks have been harvested in each region, what species are most taken, and even metrics like average birds per hunter per day. This opens the door to adaptive management within a season. For instance, if a certain waterfowl zone has a quota or objective, managers can monitor progress toward it in real time. If an emergency closure is needed (say, a sudden outbreak like avian cholera), they can notify all checked-in users immediately through the app. Conversely, if harvest is much lower than expected due to an unforeseen migration shift, that information might shape late-season regulations or habitat management actions promptly.
Benefits of Real-Time Harvest Reporting with GOVRAX
Integrating GOVRAX or similar real-time digital reporting into waterfowl harvest monitoring workflows would address many of the issues identified with current methods. Below are key benefits and improvements that such a system offers, especially when compared to end-of-season recall surveys:
Greatly Reduced Recall Bias: Because hunters log harvests and observations immediately (or at least the same day), the need to recall events from memory is eliminated. This improves data accuracy significantly. Every bird recorded in GOVRAX is one that was harvested at that time and place – no more reliance on rough season summaries or guesswork. The data is as granular as the wing survey (which identifies species/sex of each bird) but tied to the individual hunter and hunt context without needing to mail in parts. In essence, it creates a “living harvest diary” with fidelity far beyond what a post-season survey could achieve.
Higher Response and Participation Rates: By making reporting a seamless part of the hunting process (e.g., checking out of a site), compliance can approach 100% of participants on that land, instead of a small fraction responding to mail surveys. Even in cases where reporting isn’t mandatory, the convenience and immediacy of an app can yield better participation than paper forms. Push notifications and reminders can be used to prompt forgetful users (“Don’t forget to mark your harvest before you leave!”). Additionally, because hunters see direct value (e.g. personal logs, scouting info from sightings, etc.), they are more likely to engage. In short, the barrier to participation is lowered, and nonresponse bias is minimized. We move from surveying a sample of hunters to potentially capturing data from every hunter who uses the system. This turns harvest estimation into more of a census or at least a much larger sample, improving statistical confidence.
Improved Data Precision and Less Bias: With stratified sampling and recall surveys, there was always a need to weight and extrapolate, with room for errors in the weights. In contrast, real-time reporting can collect exact numbers of harvested animals tied to individual hunters. If widely adopted, this could remove the need for expansion factors entirely – you’d be tallying actual reported harvests. Even if not every hunter uses the app initially, agencies could directly compare the app’s reported harvest with traditional estimates to develop correction factors (essentially using the app data as ground-truth). Over time, as usage grows, the reliance on inflated estimates drops. Also, digital entry reduces transcription errors; no illegible handwriting or mis-keyed data, and required fields can ensure completeness (e.g., you must select a species, and cannot accidentally skip the number of birds). The data pipeline is cleaner and more automated, yielding higher-quality data for analysis.
Real-Time and Near-Term Analytics: One of the greatest advantages is timeliness. Harvest data becomes available instantly or within days, not months. Agencies could analyze in-season trends: for example, see how opening weekend compares to the second week, or how harvest shifts when a cold front arrives. This timeliness allows for responsive management. While major framework changes (like season length) typically wait until the next year, other actions can be taken sooner. For example, suppose mid-season reporting shows that a particular duck species is being harvested at unusually high levels. In that case, managers might issue advisories or increase law enforcement presence to ensure limits are adhered to. Or if reporting shows poor harvest in one zone (perhaps due to drought reducing wetlands), they might consider habitat interventions or mid-season reallocations of hunting opportunity to wetter zones. Additionally, year-end summaries could be published within weeks of season closure, allowing all stakeholders (biologists, hunters, conservationists) to discuss and learn from the data while it’s still fresh. Faster data = faster feedback for conservation measures.
Enhanced Spatial Insights: Because every data point in GOVRAX has a location, analysts can produce high-resolution maps of harvest distribution and wildlife sightings. This can reveal patterns that were previously obscured by broad state or flyway averages. For instance, managers could identify “hotspots” of high harvest that might indicate key habitats or staging areas used by waterfowl. They could also see areas with lots of hunting activity but low success, flagging potential issues (maybe those areas need habitat improvement or are being hunted at suboptimal times). On private lands enrolled in access programs, landowners could get feedback on the game usage of their property. In short, the spatial component of the data adds a new dimension to management decision-making. It also allows more direct correlation with habitat surveys (which are often geospatial) – for example, overlaying harvest locations with wetland conditions or with BPOP strata to see how well harvest pressure aligns with population abundance.
Correlation with Population & Nest Data: By accumulating wildlife sighting reports from many users, GOVRAX can help fill information gaps in periods outside of hunting season. That data, when shared with biologists, could improve nest count and brood success estimates. For example, if many spring turkey hunters record seeing duck broods on prairie potholes, that’s valuable anecdotal evidence of local nesting success that can supplement formal surveys. On a large scale, such sightings could even be used to model breeding population indices in near real-time. Imagine a machine learning model that takes habitat conditions (e.g., satellite data on wetlands), plus crowdsourced sightings of waterfowl in May/June, and predicts the fall flight, months before the official BPOP report is fully analyzed. While not replacing rigorous surveys, this could provide an early pulse on what to expect, allowing proactive adjustments. Furthermore, when the formal BPOP or banding data arrives, it can be compared against the richer context of observations that hunters and other outdoor users logged throughout the year. Essentially, GOVRAX bridges the gap between harvest data and biological data, creating a continuous feedback loop: hunters report what they harvest (outcome of population), and also what they observe (state of population), feeding that information back into conservation planning.
Reduced Administrative Burden and Cost: The current HIP survey process involves printing and mailing forms, manual data entry, and multi-step follow-ups – all of which incur costs and logistical effort. Transitioning to a digital platform like GOVRAX can save time and money for agencies in the long run. Data is collected and stored electronically at the point of origin, eliminating many intermediate steps. Analysis that used to require compiling databases from scratch each year could become as simple as querying the live dataset. Moreover, the platform can be updated or adapted as needed (for example, adding a question to all users about ammunition type used, if a particular study calls for it), which is much easier than altering a paper survey and retraining hundreds of license vendors. The Federal Register notice on improving migratory bird surveys explicitly asks for ways to minimize burden, hinting at “electronic submission” and other IT solutions – GOVRAX is precisely such a solution, aligning with agency goals to modernize data collection.
Engaged Conservation Community: Beyond the technical and scientific advantages, implementing GOVRAX fosters a more engaged relationship with hunters. Hunters become active participants in data collection and can see the role their data plays in management. The app could provide instant feedback, such as showing the user a summary of their season stats or how their reports contributed to statewide numbers. This transparency and involvement can build trust – the same way waterfowl hunters embraced decades ago the idea of mailing in duck bands and seeing band return reports, they can embrace digital reporting as part of the hunting tradition. More engaged hunters are likely to support conservation initiatives (habitat programs, regulations based on data, etc.), completing the circle of user-driven wildlife management. The GOVRAX platform’s community features (like sharing observations or viewing aggregate results) reinforce that hunters, biologists, and land managers are on the same team, united by information sharing. In the long run, this could help recruit and retain hunters by highlighting the conservation impact of their participation, a vital consideration as hunter numbers decline nationally.
Conclusion
The current system of waterfowl harvest reporting in the U.S. – built on end-of-season HIP surveys and decades-old methodologies – has provided invaluable long-term data but is showing its limitations in today’s fast-paced, data-driven world. Challenges like recall bias, low response rates, and coarse, delayed data hinder our ability to fully understand and manage waterfowl harvest. As we have shown, these limitations can lead to biased estimates (in some cases, substantially overestimating or underestimating harvest) and missed opportunities to connect harvest outcomes with real-time environmental factors. With waterfowl populations facing pressures from habitat loss and climate shifts, the need for accurate, timely harvest information is more important than ever.
GOVRAX represents a promising path forward. By leveraging mobile technology and the enthusiasm of outdoorsmen, it offers a way to revolutionize harvest data collection, making it instantaneous, precise, and rich with contextual information. Integrating GOVRAX into agency workflows would mark a shift from retrospective estimation to proactive monitoring, enhancing both harvest management and conservation science. It aligns with the USFWS’s own calls for innovation in data collection and could be implemented through partnerships and pilot programs (for example, a state could roll it out for their public hunting lands as a testbed). The platform’s features directly map to solutions for the issues identified: real-time logging solves recall error, automated check-ins boost reporting compliance, and geotagged data opens new analytical vistas.
For government agencies, conservationists, and hunters alike, the adoption of a tool like GOVRAX is a win-win. Agencies get higher fidelity data to inform decisions (from setting duck seasons to evaluating habitat projects), conservationists gain a trove of information to correlate harvest with population health, and hunters get a modernized experience where their role in conservation is visible and valued. The end result would be management strategies that are more responsive and grounded in current reality, ensuring sustainable harvests that match the true status of waterfowl populations.
In conclusion, the marriage of technology and tradition – using twenty-first-century tools to enhance a century-old conservation model – holds immense potential. By moving from paper surveys to real-time apps, we can overcome the long-standing issues of recall and reporting bias that have challenged harvest surveys. GOVRAX, founded by individuals who understand both the military precision required for missions and the passion of days spent in the duck blind, embodies this new direction. Embracing such innovation will allow us to better conserve our waterfowl resources and uphold the proud legacy of North American waterfowl management, keeping it strong for generations of hunters and wildlife enthusiasts to come.
Works Cited
Aubry, P., M. Guillemain, et al. “Attenuating the Nonresponse Bias in Hunting Bag Surveys: The Multi-Phase Sampling Strategy.” PLOS ONE, vol. 14, no. 3, 2019, e0213653.
Connelly, Neil A., and Tommy L. Brown. “Use of Estimates and Importance of Recall Bias in Hunter-Reported Harvest Data.” Human Dimensions of Wildlife, vol. 1, no. 2, 1995, pp. 57–66.
Dinsmore, Stephen J., et al. “Estimating Waterfowl Population Size Using Band Recoveries and the Lincoln Estimator.” Journal of Wildlife Management, vol. 78, no. 2, 2014, pp. 251–258.
Federal Register. Proposed Information Collection: Migratory Bird Harvest Information Program Surveys. 88 FR 6702, 2023.
GOVRAX, LLC. Internal Documentation, Company Overview, and Founder Interviews (Cassat & Neal), 2024.
Lebreton, Jean-Dominique, and P. M. Hammond. “Improving Harvest Estimates in Wildlife Management: A Review.” Ecological Modelling, vol. 402, 2019, pp. 22–34.
Padding, Paul I., and J. Andrew Royle. “Evaluation of Bias in U.S. Waterfowl Harvest Estimates.” Journal of Wildlife Management, vol. 76, no. 3, 2012, pp. 336–342, 575–584.
Raftovich, Richard V., Chandler S. Chandler, and Kevin A. Wilkins. Migratory Bird Hunting Activity and Harvest During the 2009 and 2010 Hunting Seasons. U.S. Fish and Wildlife Service, 2011.
Sen, A. R. “Response Errors in Canadian Waterfowl Surveys.” Journal of Wildlife Management, vol. 37, no. 4, 1973, pp. 485–491.
Thogmartin, Wayne E., et al. “A Comprehensive Review of Harvest Data Collection Methods for North American Game Birds.” Wildlife Society Bulletin, vol. 34, no. 4, 2010, pp. 1210–1221.
U.S. Fish and Wildlife Service. “Harvest Information Program.” U.S. Fish and Wildlife Service, www.fws.gov/program/harvest-information-program.
- Migratory Bird Harvest Surveys: Overview and Methods. www.fws.gov/birds/surveys-and-data/harvest-surveys/overview.php.
- Migratory Bird Hunting Activity and Harvest During the 2019–20 and 2020–21 Hunting Seasons: Preliminary Estimates, 2021.
- Waterfowl Breeding Population and Habitat Survey (BPOP). www.fws.gov/program/waterfowl-breeding-population-and-habitat-survey.
Wildlife Management Institute. “HIP Improvement Pilot Off to a Strong Start.” Wildlife Management Institute, Feb. 2021.
Williams, Byron K., James D. Nichols, and Michael J. Conroy. Analysis and Management of Animal Populations. Academic Press, 2002.